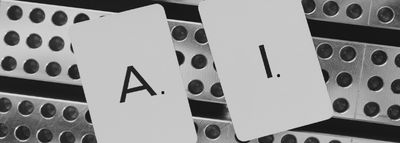
The hidden physics that sparked an AI revolution
In the mid-20th century, physicists exploring the enigmatic behaviors of spin glasses — metallic alloys with disordered magnetic orientations — could scarcely have anticipated that their theoretical investigations would later form the basis of artificial intelligence. These materials, seemingly lacking practical application, became the crucible for ideas that would revolutionize our understanding of memory and learning in machines.
In 1982, John Hopfield, a physicist intrigued by the collective behaviors inherent in spin glasses, introduced a model that reimagined memory through the lens of statistical mechanics. His Hopfield network conceptualized memories as stable states within an energy landscape, allowing for the retrieval of information by simply moving toward these low-energy configurations. This approach not only revived interest in neural networks but also bridged the gap between physics and cognitive science, suggesting that the principles governing disordered materials could illuminate the workings of the mind.
The significance of Hopfield’s contribution was formally recognized in 2024 when he, alongside AI pioneer Geoffrey Hinton, received the Nobel Prize in Physics. While some viewed this as a nod to advancements in artificial intelligence, the award underscored the profound impact of physical theories on our conceptualization of learning systems. The methodologies derived from the study of spin glasses have since become instrumental in developing neural networks capable of not only memory recall but also imagination and reasoning.
Today, as we continue to refine AI models, the legacy of spin glass physics endures, offering insights into the emergent properties of complex systems. The once esoteric study of disordered magnets has thus found its place at the heart of technological innovation, exemplifying how abstract scientific inquiry can yield transformative applications.
Source: The strange physics that gave birth to AI — QuantaMagazine